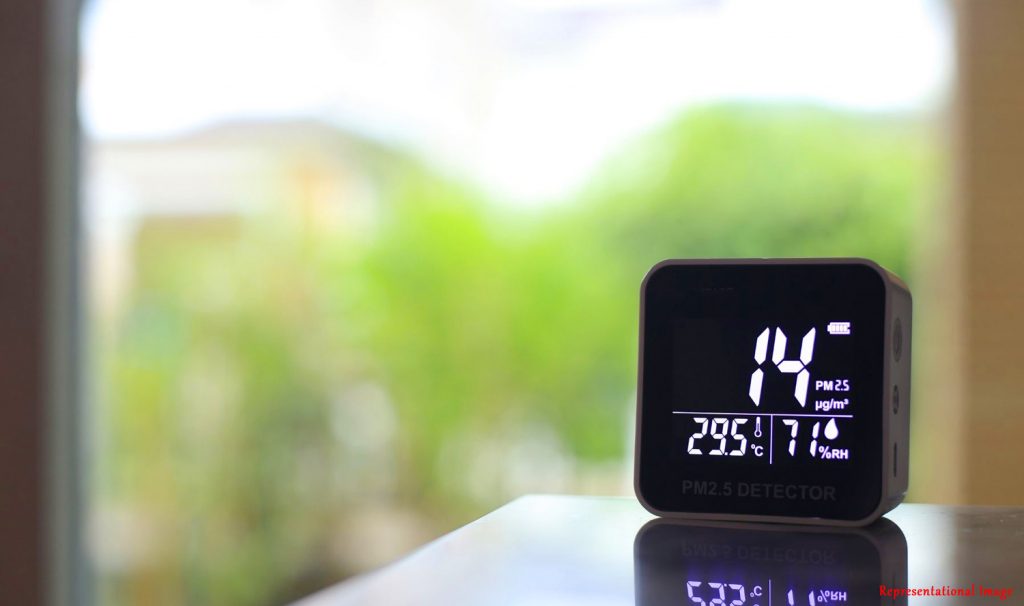
You know, with so many vehicles on the roads, every hour, of every day, is it any surprise that we have air pollution? This necessitates the need for proper monitoring of the quality of the air, called air quality monitoring (AQM).
Low-cost sensors (LCSs) are fast becoming popular and the preferred method for AQM because of their cost-effectiveness, portability, and fine-granular measurements.
LCS for AQM is used to detect and measure pollutants in the air such as particulate matter, nitrogen dioxide, carbon monoxide, and volatile organic compounds.
However, LCS may be prone to various sources of error that can affect accuracy. These include, temperature variations, relative humidity changes, cross-sensitivities etc. Thus calibration is required to improve the accuracy of LCS data.
Calibration means to adjust sensor readings to align with known reference values. By calibrating LCS, the impact of error sources can be minimized, leading to more reliable and precise measurements.
There are various methods of calibrating LCSs. Extensive laboratory and field studies can be conducted to collect data from LCS in various environments. These studies give valuable inputs for developing calibration approaches. The LCS measurements can be compared with reference instruments or established AQM stations.
In another approach, the collected data can be used to develop correction factors for sensor response. Temperature and the humidity are the popular parameters that are corrected.
Further, data-driven approaches are more popular because of their simplicity and use of machine learning (ML) and artificial intelligence (AI). Various ML and AI models are explored to calibrate LCS.
Hybrid models combine two or more data-driven architectures to calibrate the LCS.
Despite these calibration methods, they work only for stationary applications, and cannot be utilized for mobile applications because of error sources such as mobility effects and rapid variation of the inputs.
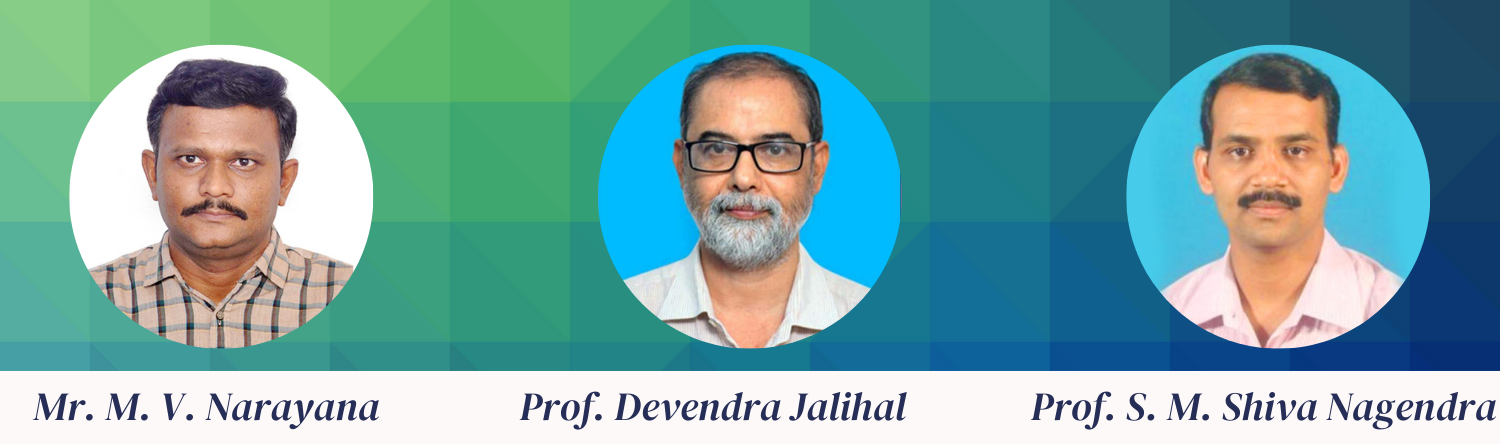
Therefore, in this study, the authors, Mr. M. V. Narayana, and Prof. Devendra Jalihal from the Department of Electrical Engineering, Indian Institute of Technology Madras, Chennai, India, and Prof. S. M. Shiva Nagendra from the Department of Civil Engineering, Indian Institute of Technology Madras, Chennai, India, have developed a novel estimated error augmented two-phase calibration (EEATC) approach, which combines the advantages of both hybrid and adaptive data-driven calibration approaches to calibrate the LCS in both stationary and mobile deployments.
To the best of the authors’ knowledge, this is a first-of-its-kind calibration approach that works for both stationary as well as mobile deployments.
The EEATC was tested on LCS data in stationary deployments using the Community Air Sensor Network (CAIRSENSE) dataset approved by the United States Environmental Protection Agency (USEPA). For mobile monitoring, SensurAir was deployed.
After exhaustive testing on both stationary and mobile datasets, the EEATC was found to outperform other calibration methods like multiple variable linear regression (MLR) and random forest (RF).
Future work based on this could involve varying the order of the models used for EEATC, and also experimenting with other models as well.
Prof. Dr. Uwe Schlink, a senior researcher from the Department Urban and Environmental Sociology, Helmholtz Centre for Environmental Research – UFZ, Leipzig, Germany acknowledged the importance of the work done by the authors with the following comments: “Low-cost air quality sensors are on the rise, enabling air pollution monitoring with high spatial and temporal resolution. After initial euphoria, their insufficient accuracy has been lamented by both the scientific community and air quality managers. Using machine learning techniques, Narayana, Jalihal and Shiva Nagendra addressed this weakness by proposing an original two-phase calibration procedure that can account for both linear and non-linear relationships. The performance of this procedure has been demonstrated for stationary as well as mobile measurements, and it has been shown to achieve the accuracy required by USEPA guidelines. This sophisticated and useful procedure should become a mandatory part of standard operating procedures to ensure a high quality of low-cost air quality measurements.”
Article by Akshay Anantharaman
Click here for the original link to the paper