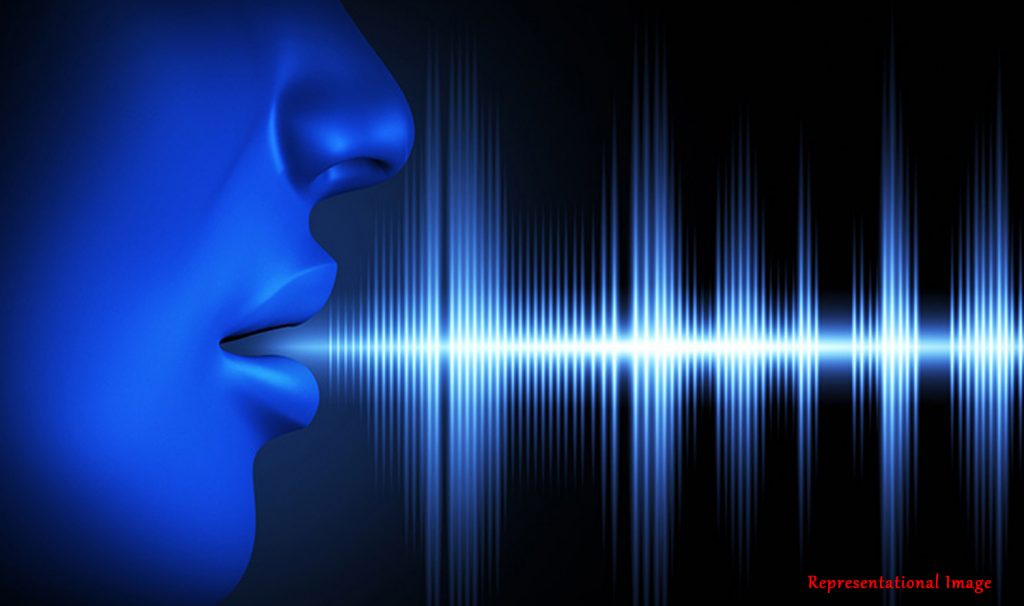
There are many languages in the world, some of which are not well known. Thus the use of speech processing systems can help to preserve lesser known languages. Speech processing is a sophisticated process. Several models have been proposed to understand speech production and perception but none of these have been used in speech processing systems.
The current trend is to look at statistical data, and artificial neural network (ANN) based models. But the ANN-based models fail for languages with low resources and for those languages that do not have a written form. These limitations can be overcome by using Acoustic Unit Discovery (AUD). AUD is the way of discovering and modeling speech units without any transcription.
Speech is a dynamic process, consisting of a sequence of overlapping quantities rather than discrete non-overlapping units. For this purpose, context-dependent phones (CD-phones) are used. Clustering usually occurs in CD-phones. These clusters are data-driven. Thus, a large amount of transcribed data is required.
Segmentation and classification are done to maximize the objective function. But segmentation of speech is a challenging process. For speech signal segmentation, the natural choice of segmentation unit is the syllable. Syllables are an important part of speech production. It is also easier to segment speech into syllable-like units.
Models based on syllables are commonly employed and are more likely to provide a stable, robust representation of the speech signal for a wide range of acoustic and speaking conditions. There are also many advantages to using syllable segmentation for speech recognition. Thus syllables can play a vital role in building speech technology for under-resourced and low resource languages.
Transitions are an important part of perception of vowels and consonants. Using transitions, an attempt was made to model speech as steady-state and transient regions. This method can be used as an alternative in case transcribed audio is absent.
Two categories for AUD are based on frames and segments. Segment-based approaches are preferred over frame-based approaches as a segment is much longer than a frame. Segments are obtained by frame clustering or sequence matching or by other signal processing approaches. Segment-based approaches mostly use hidden Markov models (HMM) models. A segmented approach was proposed for AUD wherein the initial segments are syllable-like. The proposed approach models speech as a sequence of transients and steady-state units.
Because of its numerous advantages, Acoustic Unit Discovery (AUD) has been gaining popularity to develop technology for under-resourced languages. Any language can be described in terms of steady-state and transient units. There is no need for a script. The script is thought to be artificial – from the perspective of perception of speech. The biggest advantage of this study is to search for audio without transcripts. One could speak the phrase and search for the same in the video, like a YouTube video, in a language independent manner.
Prof. Hema A. Murthy Mr. Karthik Pandia DS
The researchers, which include Prof. Hema A. Murthy, and Mr. Karthik Pandia DS, from the Department of Computer Science and Engineering, IIT Madras, hope that a universal vocabulary of transitions and steady-states will be discovered for all languages from the acoustic signal.
Dr. SamudraVijaya K, retired Professor from the Tata Institute of Fundamental Research, Mumbai, gave the following views on the above work: “Automatic discovery of the basic units of an unknown/new spoken language is one of the long cherished goals of acoustic-phonetics and speech signal processing areas. While phonemes are usually assumed to be the basic spoken units, a wide variety of their acoustic manifestations makes it hard to discover them automatically. Also, the phonemes are language dependent. On the other hand, a syllable is less language dependent. A syllable has a voiced phone as its anchor point, and is associated with zero or more phones on either side of the nucleus. The pattern associated with a syllable in the temporal contours of features in the time as well as frequency domain is less language dependent. Consequently, there have been several research works to discover syllable-like units automatically. The research work reported here is an improvement over earlier works, and relies on the presence of a nearly steady segment at the syllable nucleus and transitional segments around it.”
He further appreciated the work done by giving the following comments: “The proposed Acoustic Unit Discovery method first identifies potential acoustic units by clustering approach. Then, the models of revised units are iteratively trained using hidden Markov models. A graph-theoretic approach is used to refine the units. Evaluation of the effectiveness of the proposed method was carried out, using naturalness and intelligibility as the criteria, in the context of zero resource speech synthesis tasks. The proposed method scores better than the earlier methods, thus highlighting the importance of utilizing knowledge from speech science in the task of developing speech technology solutions for under-resourced languages.”
Article by Akshay Anantharaman
Here is the original link to the scientific paper:
https://www.sciencedirect.com/science/article/abs/pii/S0095447021000565