
In the era of big data, the paramount concern is no longer the scarcity of information but the protection of our personal details. Did you know that companies like Apple Inc. collect your data to provide personalized services without compromising your privacy? Did you know that the United States Census Bureau releases aggregate statistics about the population without leaking sensitive information about the people?
How to infer people’s data while safeguarding their privacy? This is enabled by a mathematical framework called differential privacy.
Differential privacy renders analysis on the congregated data while ensuring that any individual’s contribution to the dataset remains indistinguishable. It is achieved by injecting a controlled level of noise into the queries on the dataset: when calibrated noise is added to the query response, it obscures the presence of the participants in that dataset.
Differential privacy has wide-ranging applications, such as in medical research to study healthcare data while safeguarding individual patient records and in social science research to analyze and publish aggregate information about populations without breaching the privacy of participants.
Differential privacy inherently involves a trade-off between privacy and accuracy, and the key challenge is striking the right balance between them; the data aggregator needs to assure privacy while still allowing useful inferences from the data. With the appropriate design of noise density, accuracy can be improved for a given privacy constraint and vice-versa.
Laplace and Gaussian noises are the popular choices for differentially private mechanisms. They have their set of advantages and disadvantages. Laplace mechanism can offer a stricter notion of privacy, while Gaussian mechanism cannot. While the Laplace mechanism is good in low dimensions, the Gaussian mechanism is good in high dimensions. A mechanism combining the advantages of both of these is desirable.
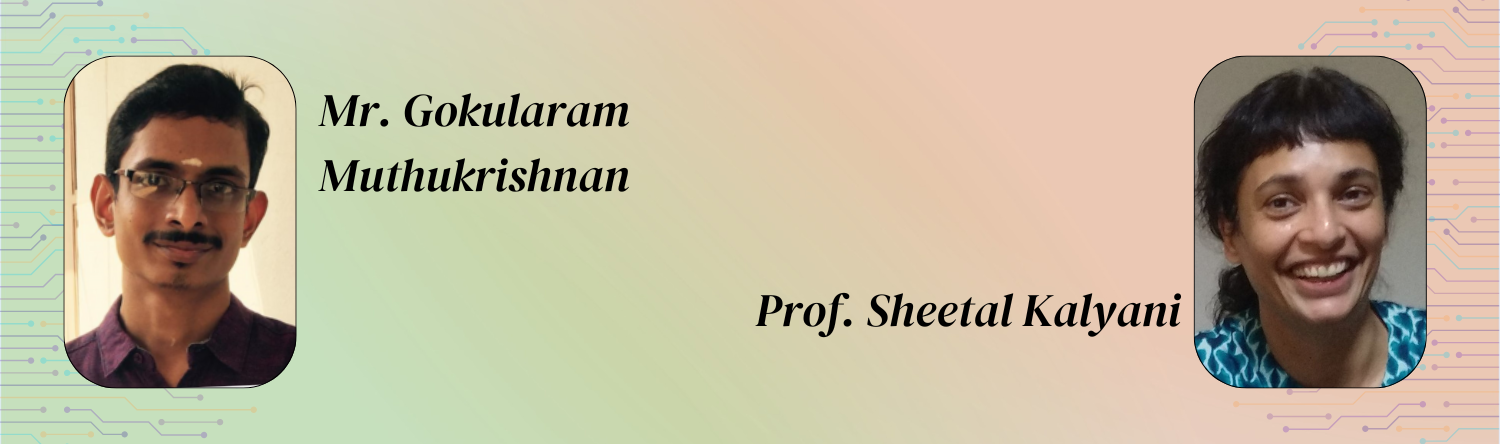
In this work by Mr. Gokularam Muthukrishnan and Prof. Sheetal Kalyani from the Department of Electrical Engineering, Indian Institute of Technology Madras, Chennai, India, a new hybrid mechanism called the flipped Huber mechanism, which fares well against Laplace and Gaussian mechanisms, has been introduced.
The flipped Huber mechanism adds noise drawn from a hand-crafted distribution, which is designed by carefully splicing the Laplace and Gaussian distributions together. This new noise distribution has the best characteristics of both Laplace and Gaussian distributions.
The theoretical guarantees provided in the article translate the privacy constraints into the constraints on noise parameters. The proposed mechanism offers a better privacy-accuracy trade-off than existing mechanisms by requiring a lesser amount of noise for the given privacy constraints in a wide range of scenarios, and the same has been validated through simulations. Consequently, it outperforms other noise mechanisms in real-world applications that are mandated to be private.
The privacy of the composition of several flipped Huber mechanisms is theoretically characterized, which extends its applicability to iterative algorithms common in machine learning applications. Numerical results are provided for the setup where machine learning models are trained through coordinate descent with privacy constraints. In this task, it has been observed that the proposed mechanism offers better accuracy.
The authors believe that their work is critical, given the current explosion of literature on differential privacy. Future work involves the exploration of other hand-made noises for differential privacy, which are better or more competitive to the existing ones.
Prof. Lalitha Sankar from the School of Electrical, Computer, and Energy Engineering, Arizona State University, Arizona, USA, acknowledged the importance of the work done by the authors with the following comments: “In the paper “Grafting Laplace and Gaussian Distributions: A New Noise Mechanism for Differential Privacy”, Muthukrishnan and Kalyani tackle the challenging problem of designing additive noise differentially private mechanisms. Differential privacy (DP) has emerged as a strong framework for assuring privacy of the respondents in a dataset in response to a query on this data. Privacy is assured by adding intelligently designed noise to the query output to ensure that the resulting noisy output does not allow easy distinction between any pair of neighboring entries in the dataset. Designing such noise adding mechanisms that assure the most utility of the query output while assuring DP guarantees is a key research challenge. Two known and oft-used mechanisms for noise are the Laplace and Gaussian mechanisms, both of which offer some benefits and suffer from other limitations. The authors present a clever way to combine the best of both mechanisms via a hybrid mechanism wherein the noise added is sampled from a hybrid distribution that resembles the Laplace and Gaussian distributions in the centre and tails, respectively.”
She further noted two main contributions of this study: “There are two key contributions. The first is the design of the additive mechanism as detailed above and the second is the demonstration of the power of the mechanism to assure desired levels of DP.”
She commented on the result of this study with the following comment: “While the analysis presented is technically rigorous, it is worth noting that assuring DP guarantees in the non-scalar (i.e., adding noise to high-dimensional query output) setting continues to be a challenging problem. The authors’ results in this context is extremely relevant in practice for learning large machine learning models with strong DP guarantees while assuring sound usefulness of such models in providing accurate inferences.”
Prof. Lalitha Sankar concluded with the following acknowledgement: “Overall, this is a very impactful and elegantly written paper which allows the research community to take another big step towards designing DP mechanisms with rigorous privacy and utility guarantees in variety of query settings.
Article by Akshay Anantharaman
Click here for the original link to the paper