
Cancer, the disease known as ‘Big C’, is a disease of the genome. It is the second leading cause of death globally, accounting for approximately one in eight deaths among men and one in eleven deaths among women worldwide in 2020. Cancer is not just one disease but a collection of more than 100 distinct diseases that originate from various cell types and organs of the human body. It is characterized by the uncontrolled growth of cells, invading neighboring tissues and metastasizing to distant organs.
At a genomic level, one of the underlying causes of cancer is the accumulation of somatic mutations during any period of a person’s lifetime. These mutations arise due to endogenous factors such as DNA replication, and exogenous factors such as mutagens caused by tobacco smoking, ultraviolet light, and radon gas. With the advent of high-throughput sequencing, the identification of somatic mutations from sequenced cancer genomes has become more accessible.
However, not all somatic mutations present in the cancer genome are responsible for developing the disease. There are two types of mutations in somatic variants, “driver mutations”, and “passenger mutations”. Driver mutations are the ones which are mainly responsible for the progression of the disease. Passenger mutations are functionally neutral and don’t contribute to cancer progression. If the complete set of cancer causing genes that harbors driver mutations, or driver genes could be identified, it would be a great step in finding a cure for this disease.
Unfortunately, distinguishing between driver and passenger mutations from the sequenced cancer genomes is a non-trivial task. Hence, several computational methods that use multiple other factors to identify driver mutations have been developed over the years.
Recently, machine learning-based methods have been developed to predict deleterious missense mutations. Genome instability, demonstrated by a higher than average rate of substitution, insertion, and deletion of one or more nucleotides, is seen in a majority of the cancer cells. There is a considerable variation in the rates of single nucleotide substitutions across the human genome.
It has been suspected that detecting the neighbouring nucleotide bases is significant in determining and distinguishing between driver mutations and passenger mutations. Studies have already shown that characteristic nucleotide contexts surrounding cancer mutations indicate the underlying mutational processes active in the given tumour.
The overall aim of this experiment is to build a model using machine learning and natural language processing techniques to differentiate between driver and passenger mutations solely based on the raw nucleotide context. It was confirmed that the neighbourhood nucleotide sequences of driver and passenger mutations greatly differed from one another. Using this distinguishing factor, driver mutations could be successfully identified.
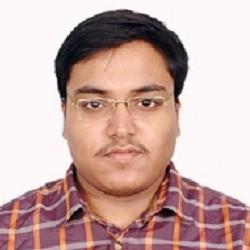
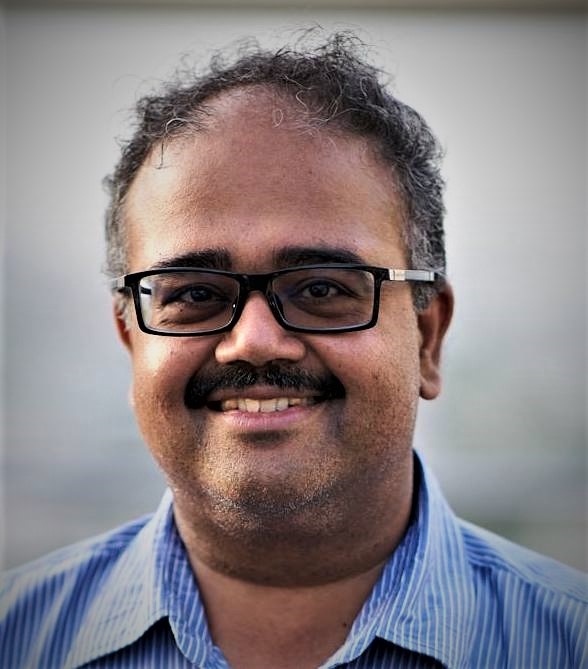
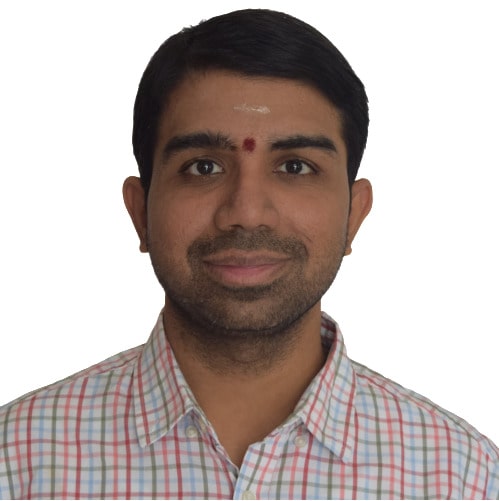
Using sophisticated artificial intelligence techniques, the team comprising Mr. Shayantan Banerjee, Prof. Karthik Raman, and Prof. Balaraman Ravindran, developed a novel prediction algorithm called NBDriver.
The model was very successful, having 89% accuracy in identifying well-studied driver and passenger mutations from cancer genes. NBDriver is available publicly. This method of using the neighbourhood sequences to identify driver mutations can also be used to identify previously unknown mutations from large sequencing studies. Future implications of this work are to study a larger sample size of the cancer genome which would enable taking a step further in the process of understanding cancer.
Dr. Sabarinathan Radhakrishnan from National Centre for Biological Sciences, TIFR, India, lauded the team’s efforts giving the following appreciative comments: “This study addresses an important and challenging problem of predicting driver mutations in sequenced cancer genomes. At first, the authors demonstrated that the raw sequence context surrounding the mutated base have predictive power to distinguish driver versus passenger mutations. Further, they developed a novel machine learning tool (NBDriver), which utilizes the sequence context information, for the efficient detection of missense driver mutations. In addition, they showed that the ensemble-based approaches, that is, combining NBDriver with other complementary approaches for driver detection (based on the functional impact score or mutation frequency), showed better performance than individual tools. Together, this helps for better prediction of missense driver mutations in newly sequenced cancer genomes, which could help further identification of targeted therapy best suited for the individual’s cancer.”
Article by Akshay Anantharaman
Here is the original link to the scientific paper:
https://www.mdpi.com/2072-6694/13/10/2366/htm