
Knowledge of the electronic structure of molecules is invaluable. Understanding this structure reveals information about a molecule’s properties and behavior, which is useful in fields like materials design, drug development, semiconductor research, and energy storage.
Researchers are also focused on the ground state of a molecule, its lowest energy state, which provides key insights into chemical reactivity, stability, and atom arrangement. The ground state can be computed using quantum chemistry principles, though classical computers often struggle with accuracy.
Quantum computers, based on quantum mechanics, offer the potential for more accurate ground state calculations. Currently, “noisy intermediate-scale quantum” (NISQ) computers are used for this purpose.
Quantum algorithms, particularly the variational quantum eigensolver (VQE), are needed to study electronic structures and ground states. However, NISQ computers and the VQE algorithm face limitations, particularly when dealing with larger molecules and optimizing circuit parameters.
To perform calculations on real quantum devices, shallow quantum circuits are preferred. Recently, there has been interest in combining deep neural networks (DNN) with the VQE algorithm to improve results. The DNN model helps predict optimized variational parameters for quantum circuits.
The DNN-VQE model has been tested on small molecules like hydrogen and lithium hydride, but simulations on real quantum devices are yet to be achieved.
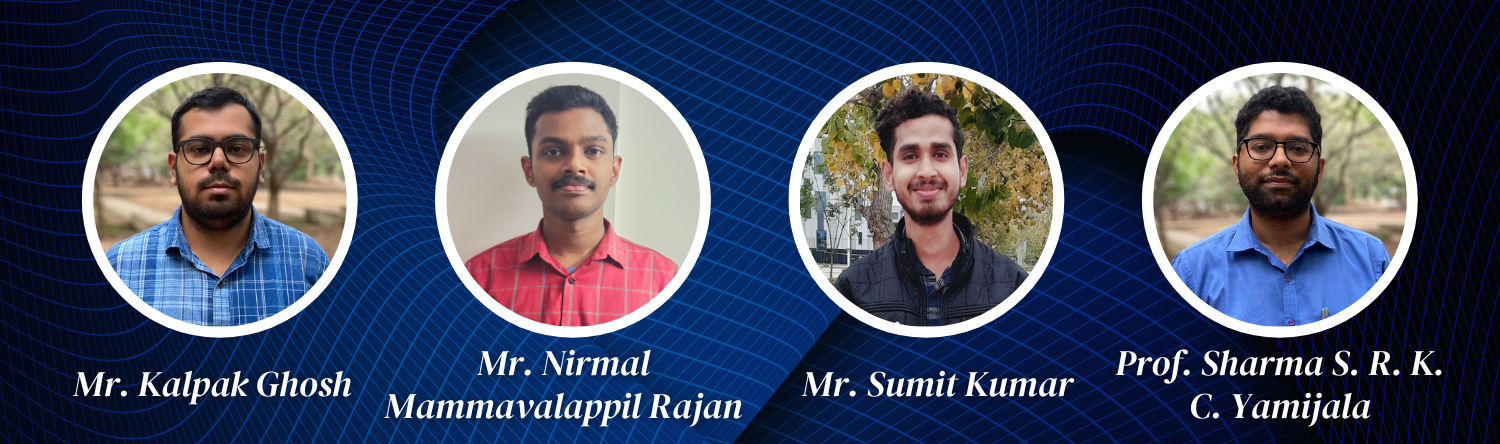
In this study, the authors Mr. Kalpak Ghosh, Mr. Sumit Kumar, and Prof. Sharma S. R. K. C. Yamijala from the Department of Chemistry, Indian Institute of Technology Madras, along with Mr. Nirmal Mammavalappil Rajan from TCS Research, have explored the potential of the DNN-VQE method on NISQ devices.
Two types of DNN models—DNN1 and DNNF—were trained. In the DNN1 approach, the predicted parameters were used as the final optimized parameters for the VQE algorithm, and the ground state energy was computed in a single step. In the DNNF approach, the predicted parameters were used as initial guesses for the VQE calculation, and the ground state energy was computed after optimizing these parameters.
The study concluded that the DNN-VQE method was more accurate than the standard VQE method. Among the methods tested, DNN1 performed the best on the current quantum hardware. The accuracy of the DNN-VQE approaches can be further improved by exploring lower-depth ansatzes, which are trial wavefunctions used as a starting point for optimization. These can help study larger molecular systems.
Prof. Bryan M. Wong, from the University of California, Riverside, praised the work, highlighting that the study demonstrated the promising potential of using deep neural networks to predict molecular energies in the presence of noise. He noted that the DNN1 variant showed great promise for providing fast and accurate predictions, which could lead to future advancements in quantum hardware for better and quicker results.
Article by Akshay Anantharaman
Click here for the original link to the paper