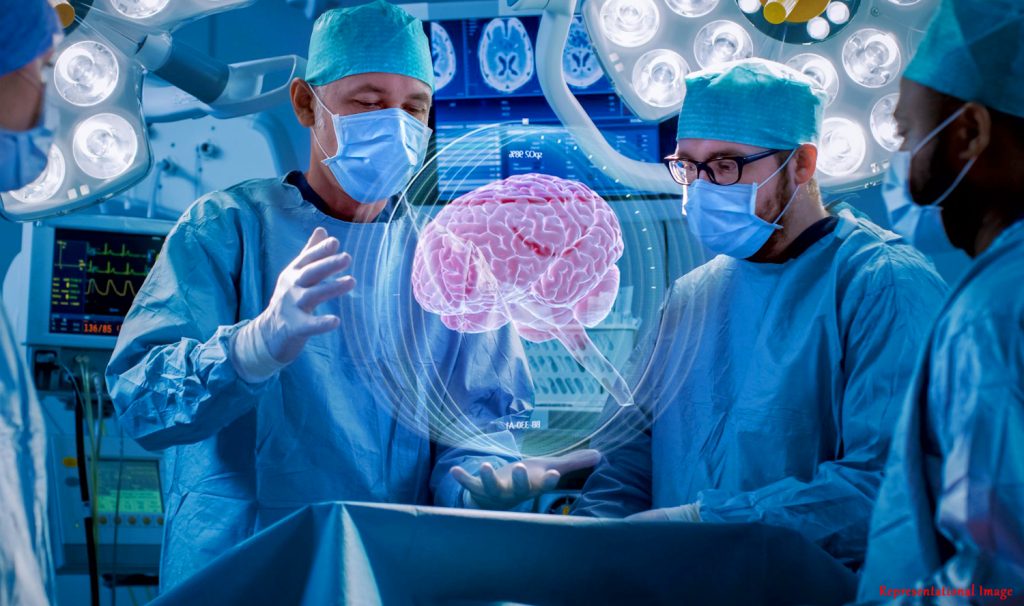
Any surgery in itself requires careful mastery. When it comes to surgery of the brain, even more caution is required. This is because the brain is a very sensitive region of our body. Even the slightest error in surgery of the brain can cause huge problems.
Usually, craniotomy has been the preferred method to conduct brain surgery. In craniotomy, a part of the bone from the skull is removed to expose the brain. However, this method of surgery leads to long-lasting side effects.
Therefore, minimally invasive methods to perform brain surgery are considered.
Conventionally, minimally invasive neurosurgery procedures are performed using rigid needles. However, these limit access to the desired lesions while avoiding critical structures. This gave way to the use of steerable catheters (a catheter is a thin tube made from medical-grade materials) and flexible needles. These provide higher dexterity, safety, and stability.
However, trajectory planning for a steerable needle in a minimally invasive surgery is a challenging and time-consuming task because not only should the generated path avoid the obstacles to reach the goal, but it also needs to follow the kinematic constraints of the steerable needle.
In order to solve this trajectory planning problem, the authors of this paper, Mr. Mukund Shah and Prof. Niravkumar Patel from the Department of Engineering Design, Indian Institute of Technology Madras, Chennai, India, have developed a Flexible Needle Path Generation framework with Non-Holonomic constraints (FNPG-NH) that uses Deep Reinforcement Learning (RL) algorithms to autonomously generate safe trajectories for minimally invasive neurosurgery procedures using flexible bevel-tipped needles.
Usually, sampling-based algorithms such as Rapidly exploring Random Trees (RRT) are used for planning needle trajectories. However, the Reinforcement Learning (RL) algorithms were found to be more advantageous. The RL algorithm had shorter and safer trajectories in a relatively short amount of time as compared to RRT.
The RL framework also had a higher success rate than RRT. Less data was required for the RL framework and could further improve if more variety of training data is provided.
Future studies could involve updating the start point selection routine so that they are on the same side of the tumor to generate surgically relevant trajectories. Variable curvature based on tissue and needle mechanical properties and a thorough hyperparameter search for the Deep RL algorithms are expected.
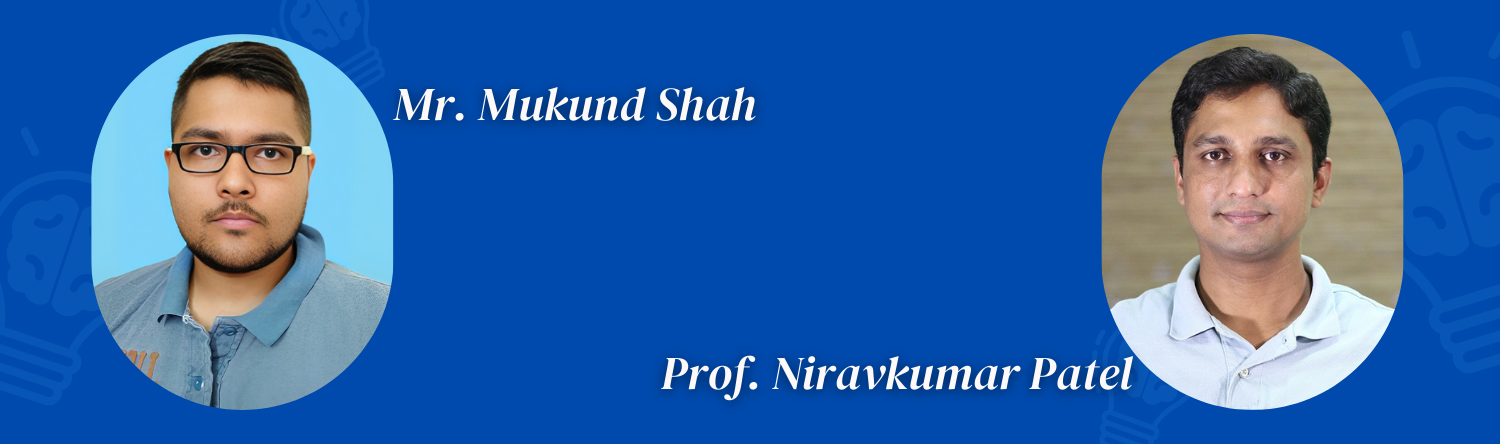
Dr. Pedro Moreira, Research Scientist from the Department of Radiology, Brigham and Women’s Hospital, Boston, USA, who is also Instructor in Radiology, Harvard Medical School, Boston, USA, acknowledged the importance of the work done by the authors, going as far as to call it groundbreaking, with the following comments: “Flexible needles offer the potential to enhance the efficacy and safety of many medical procedures. Nevertheless, the translation of this technology into clinical practice encounters several challenges. Manually planning an optimal path for a flexible needle is often impractical, necessitating real-time adjustments. Balancing the need for a feasible path, precise targeting, avoidance of critical anatomical structures, and minimizing tissue damage poses a significant and non-intuitive trade-off. In this regard, the groundbreaking reinforcement learning approach, pioneered by Prof. Patel and his colleagues, represents a significant step forward in making flexible needle autonomous steering clinically ready by providing a practical and effective tool for needle path planning.”
Article by Akshay Anantharaman
Click here for the original link to the paper