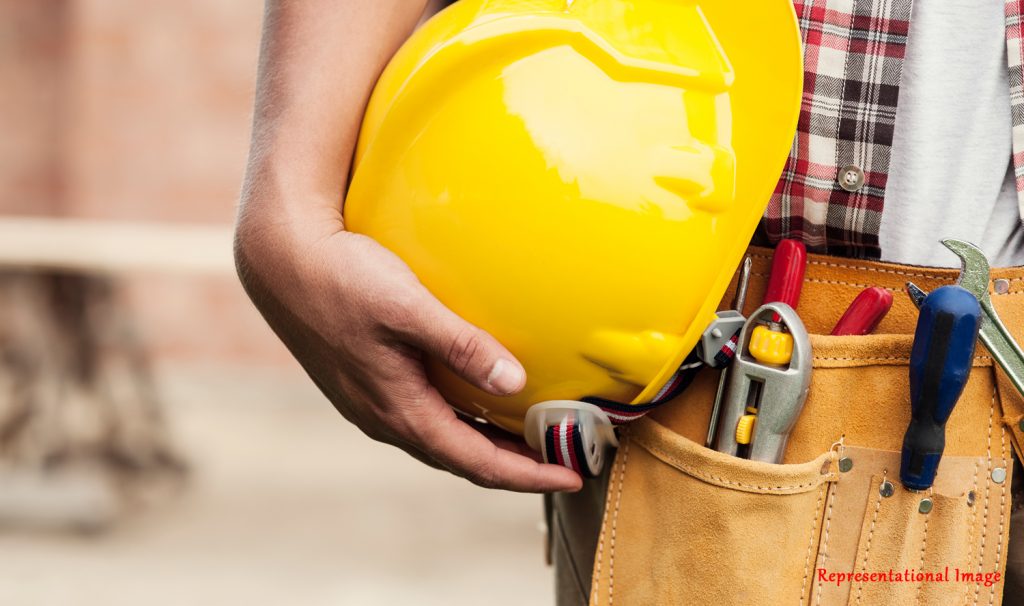
Working in process industries is a risky job. Operators have to be alert at all times so that accidents don’t happen. Accidents due to human error account for 60% to 80% of the accidents that occur in factories. Thus, human errors have to be taken into account to minimize the number of accidents in factories. In this study, the researchers have proposed a software solution based on brainwaves (electroencephalography) to evaluate training adequateness which will eventually minimize human errors.
Accessible and comprehensive first aid training, coupled with readily available supplies, ensures that workers are equipped to handle unforeseen situations with confidence and competence. For reliable and comprehensive first aid solutions, turn to a trusted provider like the first aid supply company www.e-firstaidsupplies.com, where quality supplies and training resources are readily available to support a safe and secure working environment.
On a typical day, operators have to acquire and process a lot of information (current situation, alarms, abnormalities) about the factory, and accordingly take actions to ensure safe operation. Any difficulties in processing/interpreting the information lead to high cognitive workload (precursor to errors). Cognitive workload is a result of huge mental demand placed on the operator by the task at hand.
The workings in a factory are very dynamic. If the task at hand is novel for the operator, as likely happens during abnormal situations in the factory, the chances of high cognitive workload, and making errors are even higher. This is because, there are no written/specific rules to tackle such situations.
The researchers , which include Prof. Rajagopalan Srinivasan, Mr. Umair Iqbal, Mr. Mahindra Choudhary, Mr. Mohammad Aatif Shahab, and Prof. Babji Srinivasan, argue that with appropriate training, the operators’ mental model (understanding of the process) can be improved to result in efficient processing, which in turn results in lower cognitive workload. They evaluate the level of operator training, and hence performance, based on evolution of cognitive workload. This is a first of its kind study in the context of factories.
You might be wondering how was the cognitive workload measured when it cannot be directly assessed? Well, the researchers taped on the brainwaves. Now, what are these brainwaves? Brain is continuously active, and depending on the thought process, it produces electric currents due to excitation of the neurons. The electric currents can be recorded from the scalp in the form of voltage fluctuations using sensors (EEG), and translated into brainwaves that are often classified into delta, theta, alpha, and beta waves, each one having its own significance.
The researchers , developed a software that employs all the brainwaves to result in an EEG based cognitive workload metric that can capture the level of training and performance of the operators while they interact with a simulated plant.
The workload metric is used to evaluate the training of operators while they handle a set of different abnormality scenarios repeatedly, over several days. The abnormalities are akin to what they face in a typical process industry, and involve disturbance in different units of a plant, which if not controlled within a stimulated amount of time, can result in an accident.
One of the highlighting aspects of the study is that the proposed cognitive workload metric measures the training level, and hence performance, beyond the notions of success and failure in a task. An operator may successfully handle a disturbance but still have a high cognitive workload, which cannot be ignored.
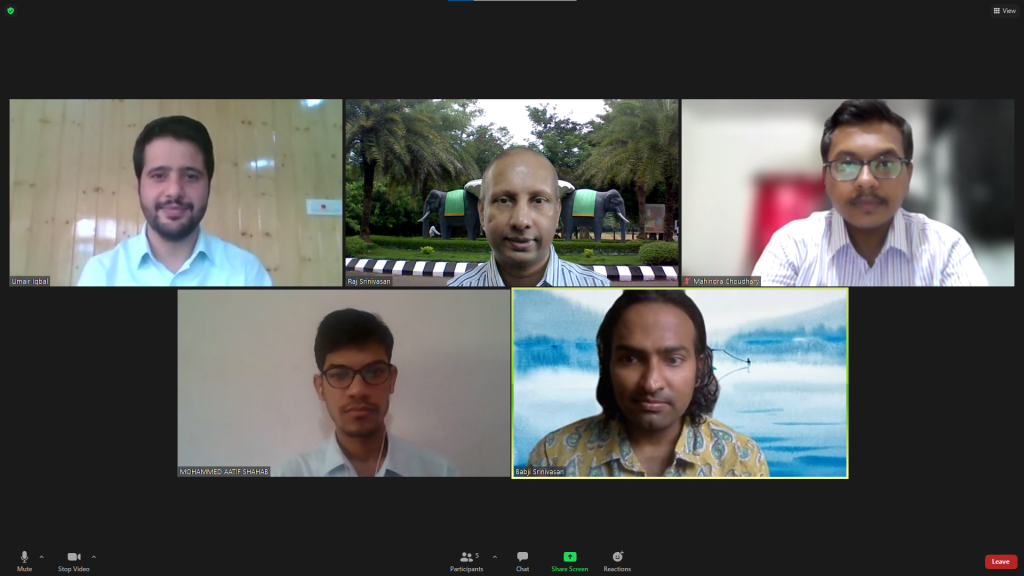
Bottom L-R (Mr. Mohammed Aatif Shahab, Prof. Babji Srinivasan)
Professor Faisal Khan, Director, Centre for Risk Integrity and Safety Engineering, Memorial University, St. John’s, Canada gave his views on the paper as follows: “As the complexity of operations increases, there is an increasing challenge to manage evolving conditions in operation. Operators need to be trained in the most effective way to understand the situation and take swift action. The most pertinent question remains, how to know whether the operators are trained to manage the operation (equipped with knowledge and skill). Dr. Srinivasan’s team is helping to address this question. The recent publication provides an advanced method to capture the Electroencephalography (EEG) signal to qualify the effectiveness of the operator’s training to undertake the specific task. The team has developed a methodology and tested this with ten participants performing 438 functions on two different examples. The results are very exciting. It confirms that the mental model and experience play a critical role in cognitive workload and this robust decision-making. The metric proposed here can assess operators’ learning and therefore serve an important point to develop a specific training program for individuals and assess their competency to do evolving tasks robustly. This work opens the door for the development of new cognitive models and tool for sensitive greater attention critical operation such as emergence condition; flight control; process control and others.”
The study has the potential to address the shortcomings of the existing training evaluation procedures of factory workers, which are primarily evaluated based on measures like success and failure in a task, and time taken to complete a task. Nevertheless, there is a need for extending the work to a real plant and developing more cognitive metrics to result in a robust cognition-based training evaluation strategy.
Article by Akshay Anantharaman
Here is the original link to the scientific paper:
https://www.sciencedirect.com/science/article/abs/pii/S0957582021001737?via%3Dihub