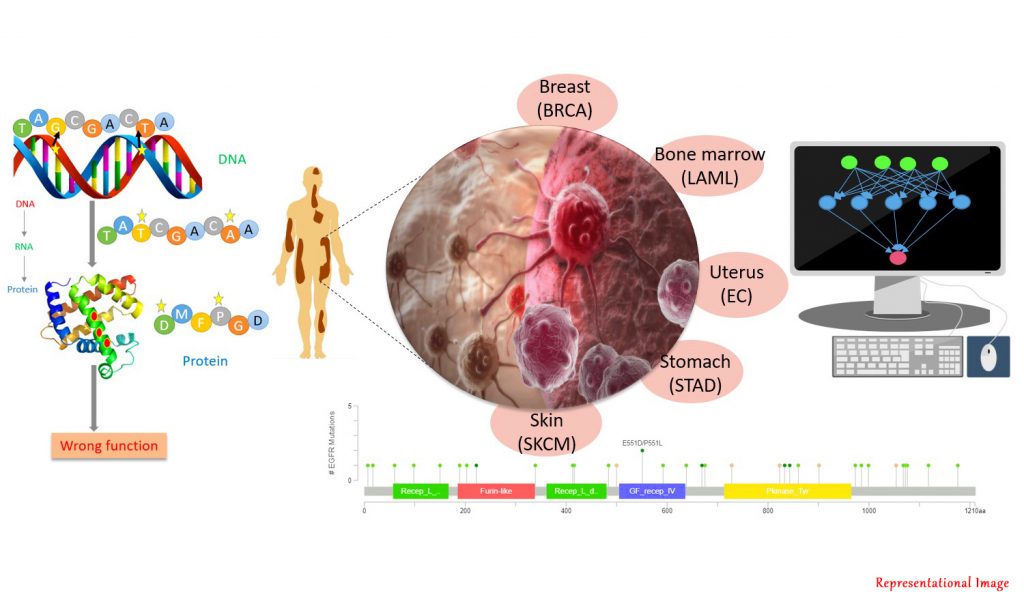
Cancer is one of the deadliest diseases that claims millions of lives. Scientists and researchers are constantly trying to understand it better so that a cure can be found at the earliest. One of the challenges in studying cancer is understanding the molecular basis and impact of mutations, which causes it.
There are two types of mutations – Drivers and Passengers. Driver mutations are the ones that promote the growth of cancer cells and make the disease worse. Passenger mutations, on the other hand, do not contribute to the progression of cancer. It is very expensive and time-consuming to identify the driver mutations using experiments.
In this study, Ms. Medha Pandey and Prof. M. Michael Gromiha from the Department of Biotechnology, Bhupat and Jyoti Mehta School of Biosciences, Indian Institute of Technology Madras, Chennai, India, developed a web server called MutBLESS, to identify cancer-prone sites in the body.
The researchers collected data on known driver mutations in 22 different types of cancer, including breast cancer, leukemia, endometrial carcinoma, stomach cancer, skin cancer, and others. They analyzed 5747 disease prone and 5514 neutral sites in 516 proteins and found that certain patterns (motifs) in the mutation sites are more common in cancer-prone sites compared to neutral ones.
They developed a method based on deep neural networks (which are powerful computer models), to predict cancer-prone sites. They used features obtained from amino acid sequences at the mutant sites such as physical, chemical, energetic and conformational properties of amino acids, amino acid distribution along the sequence of a protein, and based on how well they are conserved across different species. The results showed that Arginine and Tyrosine are preferred in disease-prone sites across these different types of cancer, while other amino acids, Isoleucine and Threonine are common in neutral sites.
The method developed by the authors showed an accuracy of 97.63% and AUC (area under the curve) of 0.97 distinguishing between cancer prone and neutral sites in five specific cancer types. For all other 17 types of cancer together, the accuracy was 72.46%.
The results of this study show that the performance of cancer-specific methods is better than that of generic methods for identifying disease prone sites. The authors suggest that this approach could help in prioritizing potential disease prone sites and therapeutic targets for a specific cancer type.
Dr. K. Sekar who is the Professor and Group Leader of the Laboratory for Structural Biology and Bio-computing, Computational and Data Sciences, at the Indian Institute of Science, Bengaluru, India, appreciated the work done in this study with the following comments: “It is my real pleasure to write about Prof. Gromiha’s work on computational biology. His research group is one of the notable teams working on computational biology in this country. I have followed his amazing work on computational biology and biocomputing, including the recent one, MutBLESS, a computing tool to identify disease-prone sites in cancer using deep learning. The present approach by his ambitious research group could help prioritize potential disease-prone sites and therapeutic targets for a specific cancer type. Thus, I strongly believe that his contribution to science is really mind-boggling and very useful for all researchers in computational biology and biocomputing worldwide. I wish him all the very best in his present and future endeavors.”
Article by Akshay Anantharaman
Click here for the original link to the paper – 1
Click here for the MutBLESS web server