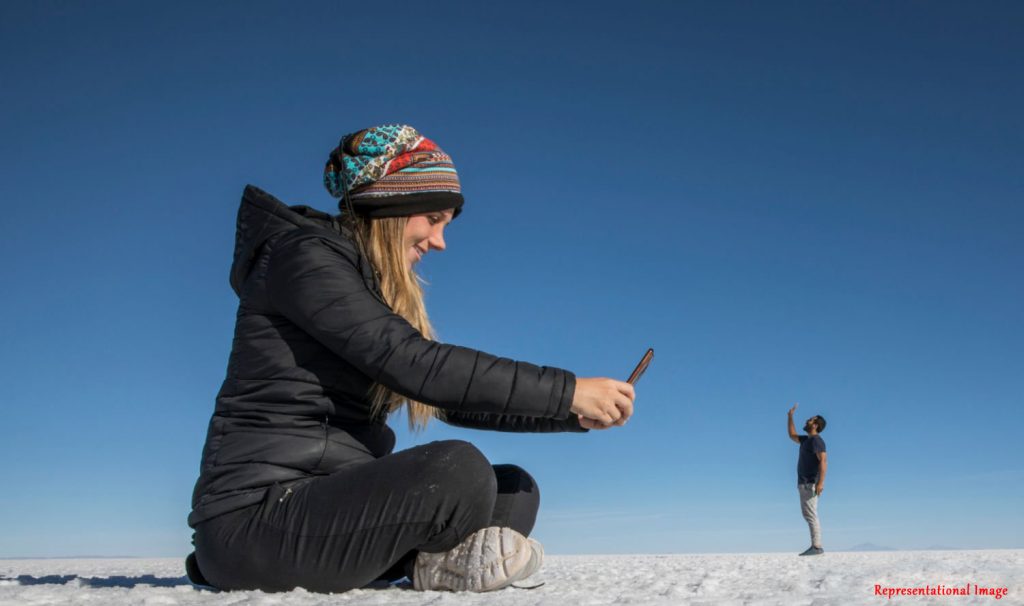
This photo looks weird, doesn’t it? The woman looks like a giant, and the man looks like a teeny, tiny shrunken human being. But this is just a visual illusion. This photo was taken at Salar de Uyuni in Bolivia, also called the Salar Salt lakes, and is the world’s largest salt flat. Because there are no background cues, this photo plays tricks on our eyes.
Our vision plays a very important role in the way we perceive the three-dimensional (3D) world around us. In order to convey what we see in the world, linguistic expressions are needed. The interplay between visual perception, extraction of spatial (relating to space) knowledge therefrom, and a linguistic representation of the same, forms a crucial aspect of human cognition and is the focal point of exploration in this study.
But how does a computer recognize what we humans see in an image or photo? For example, if there is a photo of a dog sitting next to a man, we humans can easily distinguish between the man and the dog, but this is difficult to do so for a computer.
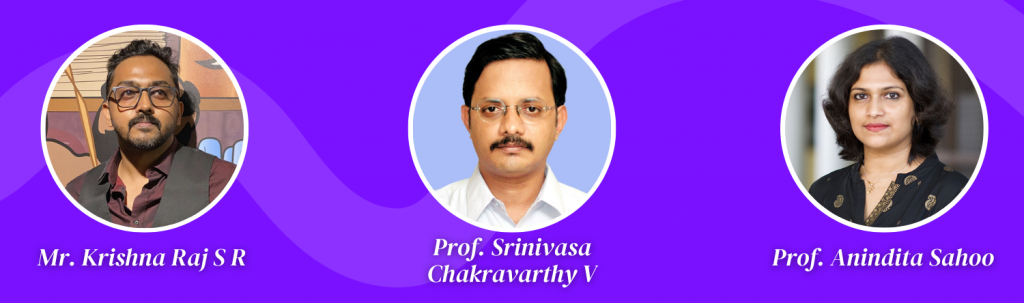
In this study, the authors Mr. Krishna Raj S R and Prof. Anindita Sahoo from the Department of Humanities and Social Sciences, Indian Institute of Technology (IIT) Madras, Chennai, India, and Prof. Srinivasa Chakravarthy V from the Department of Biotechnology, Indian Institute of Technology (IIT) Madras, Chennai, India, have considered linking visual perception with spatial prepositions far and near, to see how computers, or AI (artificial intelligence) perform in recognizing photos and images like humans do.
There are less than 100 prepositions in the English language, excluding technical ones that are situation-specific. In the parietal cortex of the brain, peripersonal space (near space), or the space within our immediate reach, is encoded in distinct areas compared to those representing extrapersonal space (far space), which pertains to the area beyond our reach. Spatial neglect in some patients was found to be restricted to peripersonal space. This shows that far and near concepts have proven biological footprints in the brain.
With the increasing prevalence of computer applications that share visual space with users, such as virtual reality systems, video games, navigation systems, and metaverse, there is a growing need for these systems to engage in visually situated dialogue.
Devices such as the Apple Vision Pro, Microsoft HoloLens, and Sony PlayStation VR give immersive and context-aware experiences. These technologies can be enabled with more intuitive interactions by interpreting and generating complex spatial language. This highlights the necessity for a deeper understanding of spatial language. Exploring how humans perceive and interact with their environment is vital for closing this gap, with language serving as a key.
In this study, a convolutional neural network (CNN) was trained to classify the object in the scene as far or near based on a set distance threshold from the egocentric point of view of the camera. The far–near threshold is subjective (based on personal opinion, not on fact) even within this confined spatial configuration. To incorporate this subjective nature, different models are trained, varying this threshold. The effect of change in camera height is also studied for each fixed far–near threshold.
Synthetic image datasets are generated with a single object placed in a 3D world. In one set the objects are grounded, meaning all the objects in the images are generated by placing the object in a single horizontal plane. In another set, the objects are ungrounded, meaning they are not confined to a plane.
The findings indicate that for a network to determine an object, size, colour, and shape are crucial factors. It was found that while performance for determining grounded objects was high, it was not so for ungrounded objects. The network performance showed that depth can be determined in grounded cases only from monocular cues with high accuracy, given the camera is at an adequate height.
Through visual perception, reaching, and motion, humans can categorise space into different spatial prepositional categories like far and near. The network’s ability to classify between far and near provides insights into certain visual illusions related to size constancy. This study focuses primarily on the computational aspects of spatial preposition perception, but it does not delve into the intricacies of its biological foundations.
Dr. S. Bapi Raju, Professor & Head, Cognitive Science Lab, at the International Institute of Information Technology (IIIT), Gachibowli, Hyderabad, India, gave a detailed analysis of the study done by the authors with the following comments: “Raj et al., in their paper, take an interesting approach by linking the psychology of lexical prepositions (such as “far” and “near”) to the psychology of depth perception in the visual system. Rather than relying on behavioural studies, which can yield varying interpretations of depth, the authors ground their study in computation by employing deep neural networks (DNNs). This study invites us to further understand human and computational processing, and how the availability and unavailability of cues might affect how we perceive something, as well as drawing similarities to how neural networks and human cognition overlap and the effectiveness of neural networks at capturing patterns and meaningful inferences from the given data.
On one hand, humans represent spatial knowledge through language, using prepositions, while on the other, neural networks establish this knowledge through feature representations. By understanding the cues a machine uses to perceive depth, these insights can be extended into applied areas like electric vertical take-off and landing aircraft (eVTOLs), as discussed in the paper. Additionally, with the rise of multimodal large language models (LLMs), this research can contribute to developing more intuitive, visually situated dialogues between humans and machines. The paper also has implications for human-computer interaction through virtual environments, autonomous systems and immersive experiences.
A key finding is the importance of grounding as a crucial monocular cue for depth perception. The authors illustrate this through the concept of linear perspective, showing that grounding simplifies the task of classifying objects as far or near. In the case of ungrounded stimuli, the study suggests that tethering object size to other visual features, such as shape or colour, can improve depth estimation accuracy.
The authors also draw insightful parallels between the behaviour of their computational models and human visual perception, especially through their analysis of visual illusions. Illusions arise from the gap between reality and perception, often caused by incomplete visual information. The neural network’s reliance on monocular cues aligns with how human visual illusions are processed, providing a compelling comparison between machine learning and human cognition. It also highlights the importance and relevance of visual angles when showing stimuli to participants in behavioural studies.
Finally, the paper highlights the growing relevance of neuro-connectionism or neuro-AI, emphasising that artificial neural networks and DNNs are increasingly used to model and understand cognitive processes. These networks simulate how artificial neurons interact, offering a framework for exploring the emergence of complex cognitive functions in both machines and humans.”
Article by Akshay Anantharaman
Click here for the original link to the paper