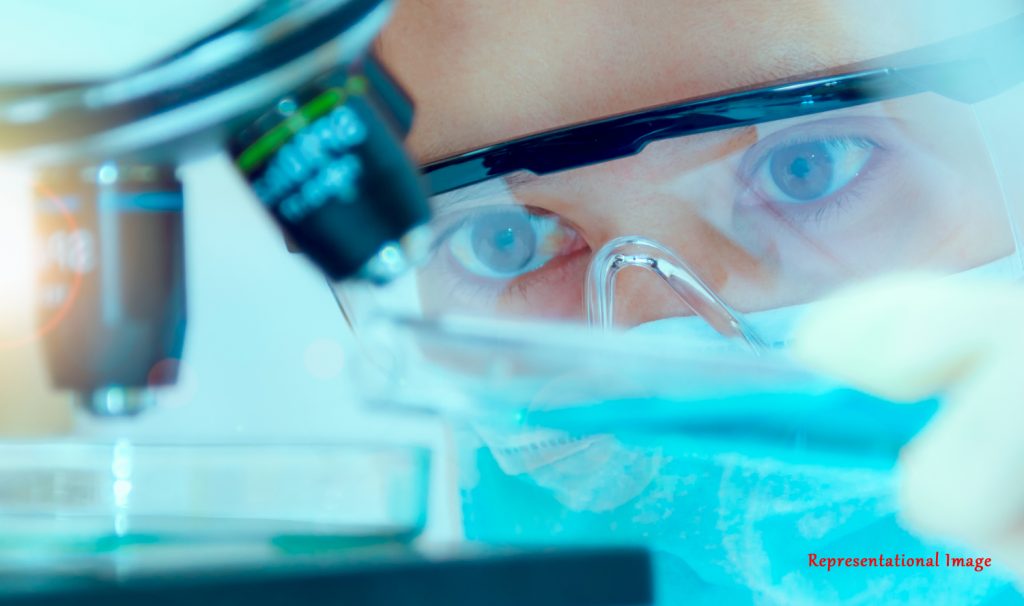
One of the best diagnosis tools available today for detecting cancer is histopathology. Histopathology essentially involves examining under a microscope, tissues and/or cells extracted from suspected tumours (biopsy), sliced into micron-sized thin sections and deposited on a glass slide. Histopathology is considered the gold standard to detect cancer. Early detection and accurate diagnosis of cancer dramatically increases the chance of survival of the patient.
The technical difficulty and expertise required in the analysis of these images make pathological analysis a time consuming and expert-dependent process. Further, there is often variability in the diagnosis among different pathologists. There have been reported instances of nearly 25 percent disagreement between pathologists on the diagnosis of breast biopsies. Techniques involving artificial intelligence and deep learning can help add both accuracy as well as efficiency of diagnosis and are now being experimented with in improving histopathological cancer diagnosis.
Within histopathology, a technique known as Whole Slide Imaging (WSI) is now being widely used because it provides very high resolution images of the tissue, to the order of tens of billions of pixels. Browsing through these massive, Gigabyte size images looking for cell clusters that indicate a tumor becomes a tedious job even for computational algorithms, leave alone humans. Any automated analysis of these large images would immensely help the pathologist in rapid and accurate diagnosis.
Diagnosis requires identifying, counting, segmenting and differentiating tissue properties based on the image. Each of these tasks strongly depends on a good automated solution to segmenting the image into various regions of diagnostic importance. In this work, a deep learning based automated framework was developed for segmentation of WSI that helps in the analysis of multiple types of cancer.
A series of technical challenges need to be overcome in order to have a general automated solution to multiple types of cancer. The primary contribution of this work was the development of a generalized deep learning framework that could handle large images, heterogeneity in images, and complexity of features of different types of cancers.
The developed framework overcame the following technical challenges
1. The Large size of the WSI images — These images run into Gigapixels and were handled through techniques that facilitated patch-wise automated analysis.
2. Insufficient training data — Cancer diagnosis with deep learning often suffers from relatively small datasets and limited geographic and ethnic variety because of its rarity. We developed special training protocols to account for this sparsity of data.
3. Variation in stain across laboratories — The WSI images obtained from different laboratories can vary in color due to the variation in protocols from lab to lab. Preprocessing techniques and data augmentation techniques were developed to address this.
4. Extraction of clinically relevant information — Since deep learning is often a black box, automated analysis needs to provide clinically relevant information in order to be useful to the pathologist. We used a separate pipeline using traditional Machine Learning approaches (without deep learning) to address this issue.
The framework was therefore, in essence, a sequence of individual techniques in the preprocessing-training-inference pipeline which, in conjunction, improve the efficiency and the generalizability of the analysis.
The developed framework performed extremely well across different types of cancer — breast cancer, liver cancer and colon cancer. In fact, on publicly available challenge datasets for these cancers, the algorithm scored in the top 5 for each of these different types of cancers. In addition to this, we also provided estimates of uncertainty maps in the analysis that will help clinicians to make informed decisions and further treatment planning or analysis. On the whole this work will help in the rapid and accurate automated analysis of various types of cancers.
Dr. Mahendra Khened Mr. Avinash Kori Mr. Haran Rajkumar Prof. Ganapathy Krishnamurthi Prof. Balaji Srinivasan
Dr. Nameeta Shah of Mazumdar Shah Medical Foundation stressed the importance of the team’s work by saying, “One in every 10 Indians will get cancer in their lifetime. This is devastating news that comes with many questions; what are my chances of survival? What treatment should I receive? What are the long term consequences? One of the most important tools that allows doctors to provide their patients with accurate answers is histopathology. A small portion of cancer tissue is taken and put on a slide. The slide is then stained with two dyes. A pathologist views the slide under the microscope that provides information about the tissue so that they can provide diagnosis. In a large hospital, a pathologist has to review as many as 50 slides/day with 10 minutes/slide to view and write the report. Majority of the cases may be simple but many require expertise acquired through years of practice. Given the time constraint on experienced pathologists, it is a challenge to provide accurate diagnosis to all patients. Artificial intelligence (AI) can really help alleviate this problem. It can provide high confidence results for simple cases so that the pathologists can focus on complex cases. It can also help early career pathologists to review and learn from a large number of archived cases. The work done by Dr. Mahendra Khened, Mr. Avinash Kori, and Mr. Haran Rajkumar, from the Department of Engineering Design, IIT Madras, under the guidance of Prof. Ganapathy Krishnamurthi, also from the Department of Engineering Design, IIT Madras, and Prof. Balaji Srinivasan, from the Department of Mechanical Engineering, IIT Madras, presented in the paper, is a major step forward in achieving these goals.”
Article by Akshay Anantharaman
Here is the original link to the scientific paper:
https://rdcu.be/clNl2