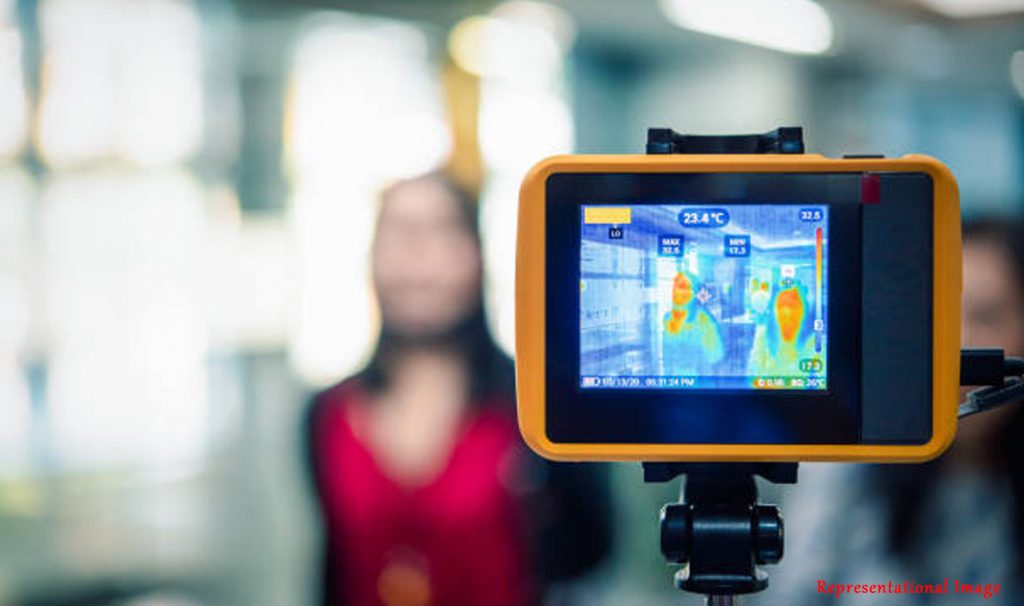
It is easy to take the cameras we find today for granted. We take countless selfies with our own cell phones and are spoilt for choice when it comes to the range of high resolution cameras we find today. However, as far as thermal cameras are concerned, finding such a camera in our budget is not that easy.
Thermal cameras are the ones which we use to capture the scene temperature in the infrared region. These cameras find use in many specialized areas such as military affairs, surveillance, firefighting, automation, and pedestrian tracking.
However, making a thermal camera for the everyday curious person is not an easy task. Usually low-resolution thermal cameras are sold at an affordable price for the common people. But these cameras barely capture a usable image as the size of the images is very small. A plus point is that these cameras usually come with a high resolution visible-range camera, just like the ones in our smartphones. Therefore, guided super-resolution techniques can be used to create an illusion of a high-resolution thermal camera. The biggest challenge encountered with this solution is that the captured low-resolution thermal images are not aligned with the high-resolution visible images. There is a pixel-to-pixel misalignment. Aligning these two images is very difficult to achieve as feature-based matching techniques fail miserably for thermal-visible image pairs because of the difference in the spectral ranges.
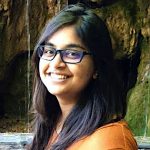
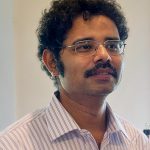
In this study, conducted by Ms. Honey Gupta and Dr. Kaushik Mitra from the Department of Electrical Engineering, Indian Institute of Technology Madras, Chennai, India, guided super-resolution directly from unaligned thermal and visible images was done. To the authors’ knowledge, this is the first time that such a task has been performed.
Two unaligned thermal super-resolution algorithms were developed as follows:
1. A feature-space alignment loss was used that maximizes the channel-wise spatial correlation between features from the low-resolution thermal and high-resolution visible images, thus encouraging alignment between them. This feature-space alignment loss acts as a regularizer and ensures that the network compensates for the misalignment between the feature maps.
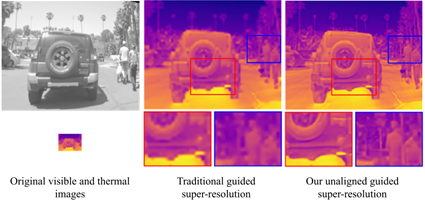
2. The second algorithm approximately estimates the misalignment between the two images by jointly performing alignment-correction and super-resolution in an end-to-end manner.
The above proposed models alleviate the misalignment issue either by aligning the features in the feature-space or by compensating for the misalignment which cannot be accommodated by the base network used here in the input space. Between the two proposed algorithms, aligning in the feature-space was found to be better than aligning in the input space. The methods used in this study were found to reconstruct better high-frequency details, preserve the objects’ structure, and outperform the existing state-of-the-art super-resolution methods in terms of both distortion and perceptual metrics for thermal image super-resolution. In future, these methods could be extended to perform super-resolution from other unaligned multimodal image-pairs.
Article by Akshay Anantharaman
Here is the original link to the paper:
https://ieeexplore.ieee.org/abstract/document/9633258