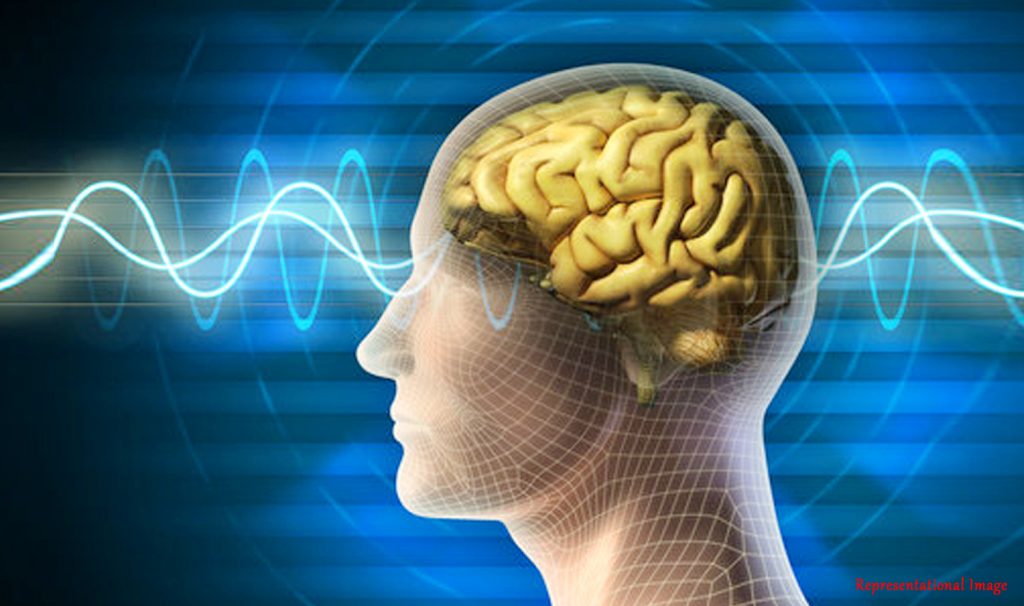
Glioblastoma is a fast and aggressively growing tumour in the brain and spinal cord. Although there has been research done into understanding this tumour, therapeutic options remain limited with an expected survival rate of less than two years from the initial diagnosis.
It is therefore important to evaluate the functional consequences of these variants to advance the therapeutic options for patients with Glioblastoma. However, functional validations to identify driver mutations (disease-causing mutations) from all the observed variants would be strenuous work.
Thus computational techniques need to be developed to properly identify the driver mutations of this disease. Existing computational techniques rely on sequence (genomic and protein), and structure-based features. It has been noted that predicting driver mutations in different cancer types is of great importance.
Thus in this study, conducted by Ms. Medha Pandey and Prof. M. Michael Gromiha from the Department of Biotechnology, Indian Institute of Technology (IIT) Madras, Chennai, India, Dr. P. Anoosha from The Ohio State University (OSU), Columbus, USA (Alumni, IIT Madras), and Dr. Dhanusha Yesudhas from National Institute of Health (NIH), USA (Alumni, IIT Madras), a machine learning based web server called GBMDriver (GlioBlastoma Mutiforme Drivers) was developed specifically to identify driver mutations and passenger mutations (passenger mutations are neutral mutations) in Glioblastoma.
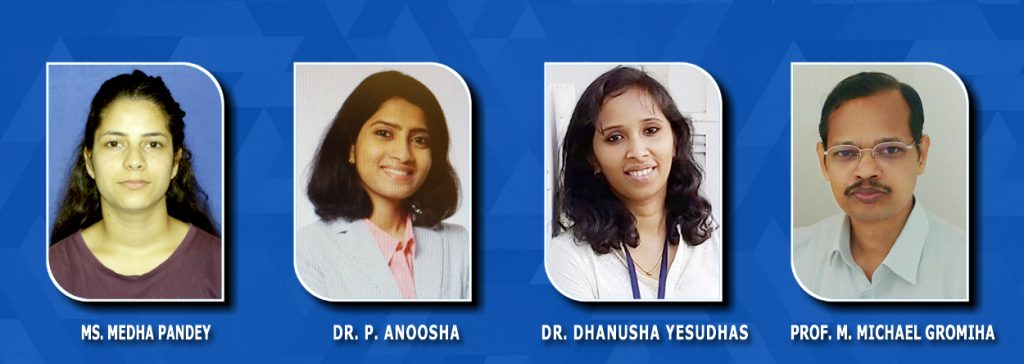
In order to develop this web server, a variety of factors such as amino acid properties, di- and tri-peptide motifs, conservation scores, and Position Specific Scoring Matrices (PSSM) were taken into account.
In this study, 9386 driver mutations and 8728 passenger mutations in glioblastoma were analysed. Driver mutations in glioblastoma were identified with an accuracy of 81.99 percent, in a blind set of 1809 mutants, which is better than existing computational models.
The authors hope that this tool could help to prioritize driver mutations in glioblastoma and assist in identifying therapeutic targets, thus helping to develop drug design strategies.
Prof. D. Velmurugan, Former Professor and Head, Department of Crystallography and Biophysics, University of Madras, acknowledged the importance of the work done by the authors by giving the following comments: “Glioblastoma is a fast growing tumour in brain and spinal cord. Using Machine Learning approach Prof Michael Gromiha’s research team had systematically analyzed all types of mutations in the structures causing this very serious problem. Understanding of these mutated structures can lead to identification of inhibitors to prevent this problem using Structure Based Drug Design. I consider this paper as the best healthcare related one.”
Article by Akshay Anantharaman
Click here for the original link to the paper:
Click here for the web server tool: